Using electronic health record data: a machine learning approach with a 2-year horizon
In the United States, end-stage kidney disease (ESKD) is responsible for high mortality and significant healthcare costs, with the number of cases sharply increasing in the past 2 decades. In this study, we aimed to reduce these impacts by developing an ESKD model for predicting its occurrence in a 2-year period.
Lead Author: Panayiotis Petousis, PhD
End-stage kidney disease (ESKD) poses a substantial burden for mortality rate and healthcare costs in the United States. We developed and
evaluated a machine learning (ML) model for predicting ESKD in 2 years using electronic health record (EHR) data. Various models were tested
by leveraging EHR data and employing an ML pipeline. The developed model outperforms existing kidney failure models. Through a chart
review, expert nephrologists affirmed the clinical utility of the model in predicting the outcome of complex cases. This model has been successfully
integrated into our academic institution as part of a dashboard with visualizations and explainability for the model’s predictions. In conclusion,
the developed ESKD prediction model demonstrates the ability to identify individuals at risk for ESKD. Any future reduction in mortality
and healthcare costs would showcase the effectiveness of our model.
Key words: machine learning deployment; early prediction ESKD model; electronic health record; end-stage kidney disease (ESKD).
Share this Article with others
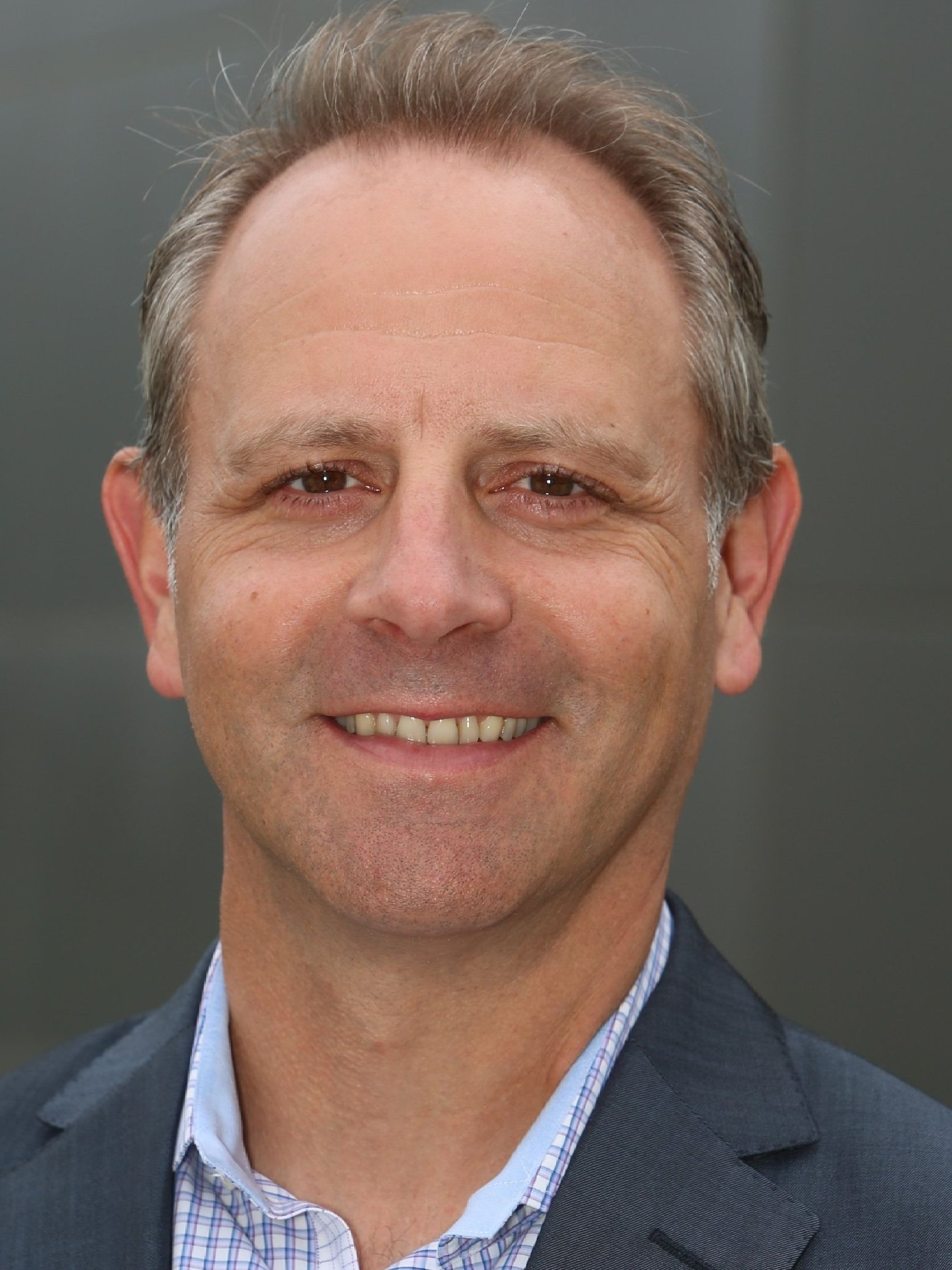